NormNets
Learning the integral connectional template of the brain
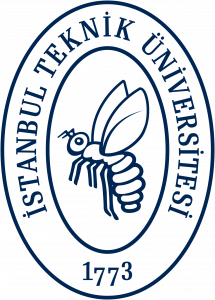
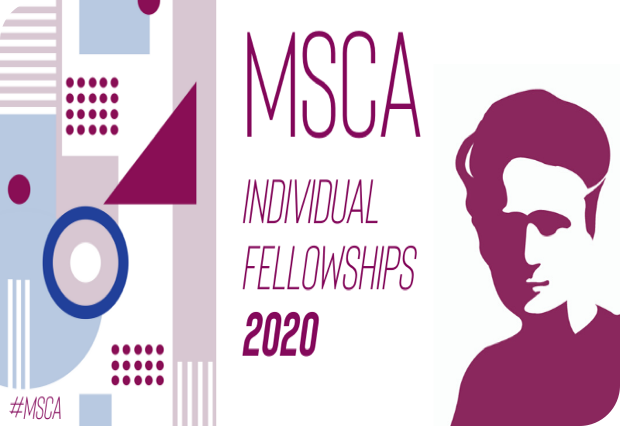
Achievements
Check out our recent NormNets publications, videos, open source codes and more ~
24 May 2020
step 0
This is the first work on estimating a connectional brain template of a population of multi-view brain networks.
netNorm can be used to integrate a population of multi-view network datasets with heterogeneous distributions, given that they have the same size.
Source code in Matlab: https://github.com/basiralab/netNorm
Source code in Python: https://github.com/basiralab/netNorm-PY
Publication: https://www.sciencedirect.com/science/article/pii/S1361841519301070
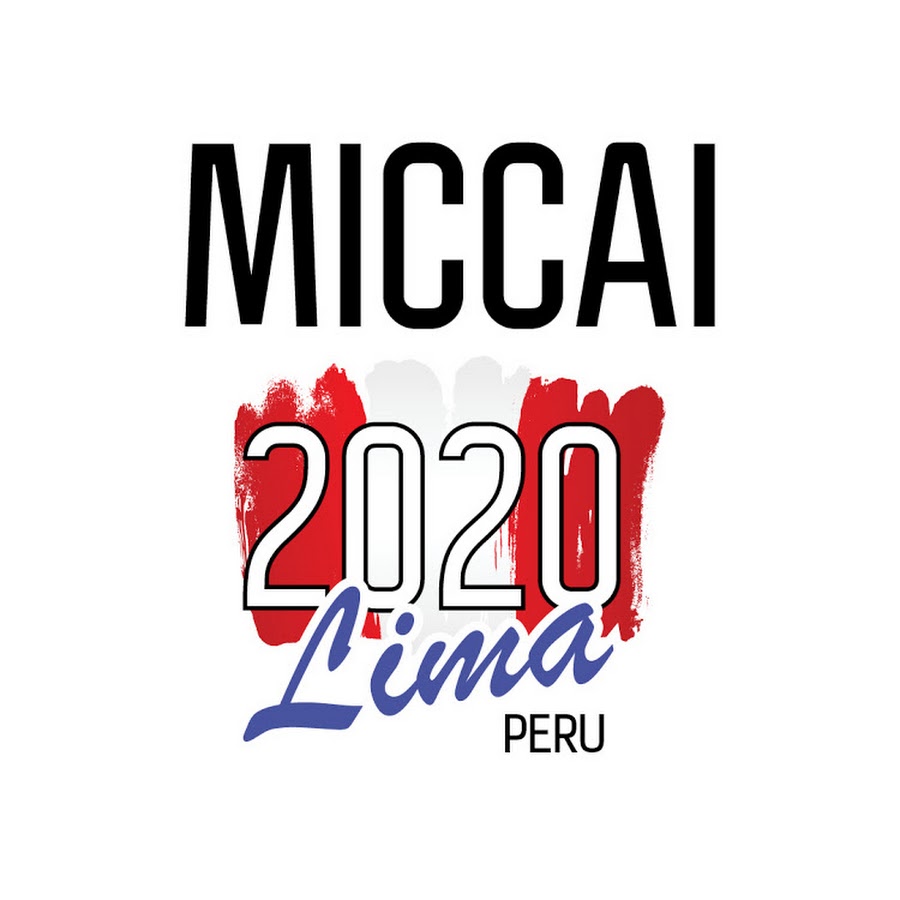
23 June 2020
Output 1
The first geometric deep learning model for multiview brain network integration and connectional brain template estimation is now accepted for publication in MICCAI (class A1) conference Springer proceedings.
Publication: M.B. Gurbuz and I. Rekik. Deep Graph Normalizer: A Geometric Deep Learning Approach for Estimating Connectional Brain Templates. MICCAI (2020), Lima, Peru (acceptance rate ~30%). —in press
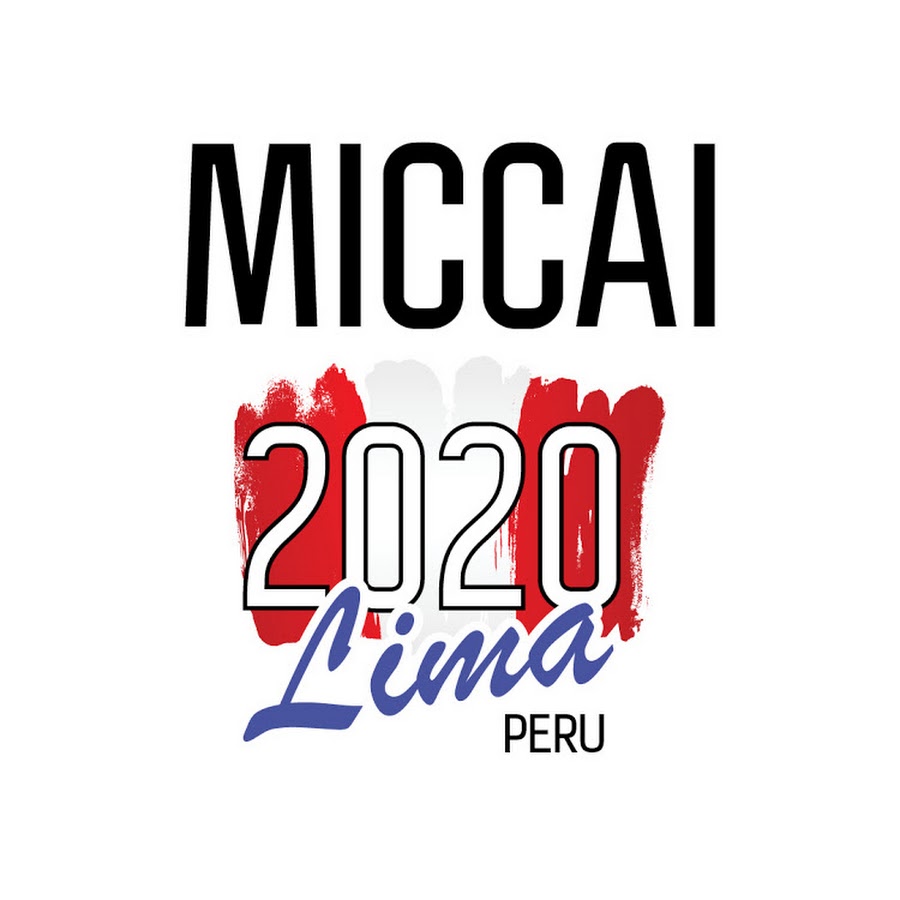
23 June 2020
Output 2
Our work on supervised brain multigraph diffusion and fusion for estimating population-driven connectional brain templates is now accepted for publication in MICCAI (class A1) conference Springer proceedings.
Publication: I. Mhiri, M.A. Mahjoub, and I. Rekik. Supervised Multi-topology Network Cross-diffusion for Population-Driven Brain Network Atlas Estimation. MICCAI (2020), Lima, Peru (acceptance rate ~30%).
check out more BASIRA lab publications
Acknowledgements: This project has received funding from the European Union’s Horizon 2020 research and innovation programme under the Marie Skłodowska-Curie grant agreement No 101003403.
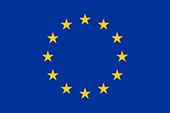
Feel free to contact us at basiralab@gmail.com to apply for positions at BASIRA lab or establish synergetic collaborations!