Brain gliomas
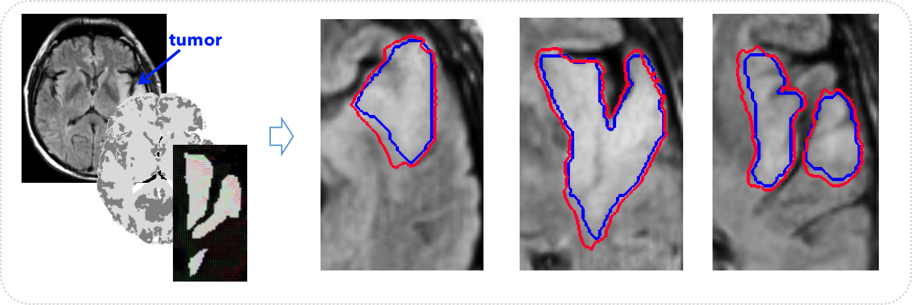
Brain glioma growth prediction from single timepoint
Motivation: Devising accurate brain tumor segmentation tools as well as predictive models of brain gliomas growth can help neurosurgeons in patient treatment.
Innovation: We develop individual-based and population-based learning methods for automatically labeling different tumor tissue types and for predicting its evolution from a single timepoint
Brain stroke
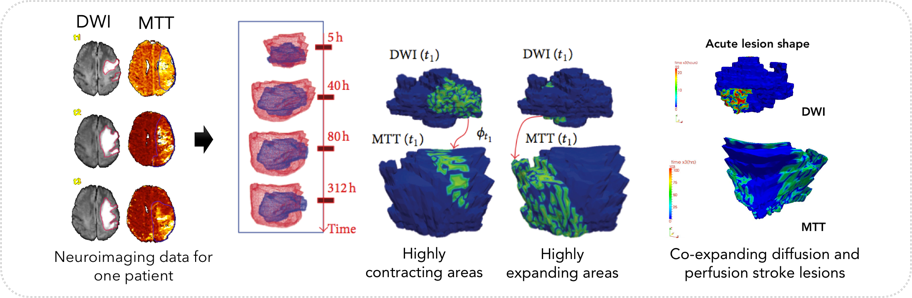
Modeling the spatiotemporal evolution of stroke lesions
Motivation: Treatment of acute stroke is based on the idea that there is salvageable tissue in the lesion. Diffusion abnormality represents the irreversibly damaged lesion core. Perfusion abnormality represents the reversible penumbra. How does an acute ischemic diffusion stroke lesion grow in relation to the perfusion lesion?
Innovation: We develop tools for modeling the 4D deformation of perfusion and diffusion lesions.
Neurodegenerative disorders
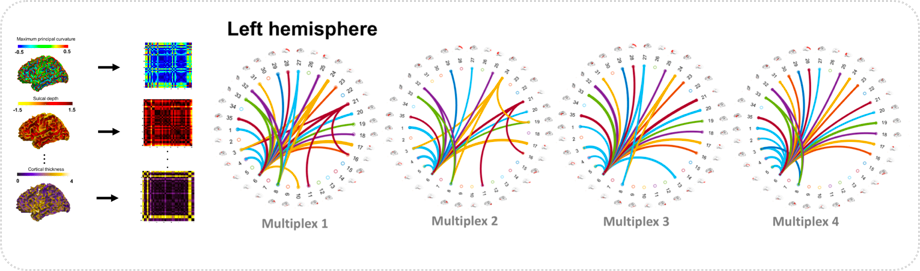
Brain morphological multiplexes for dementia diagnosis
Motivation: The accurate and early diagnosis of neurodegenerative disorders such as early mild cognitive impairment (eMCI) is essential for early patient treatment as it might be possible to slow down the progression towards a worst stage of the disorder (e.g., Alzheimer’s disease).
Innovation: We develop advanced tools for accurate and early diagnosis of brain disorders using non-invasive neuroimaging facilities (e.g., Magnetic Resonance Imaging).
Neurodevelopmental disorders
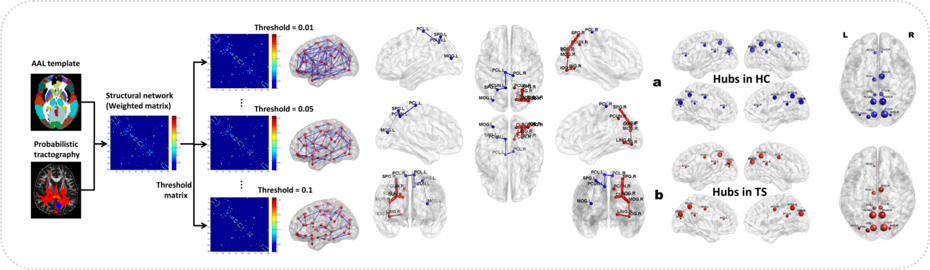
Disrupted Topological Organization of Structural Networks in Tourette Syndrome Children
Motivation: Advancing our understanding of how a specific neurodevelopmental disorder (e.g., Tourette Syndrom) affects brain functional and structural connectivity in an early stage may help identify the best treatment strategies.
Innovation: Developing machine-learning and brain network analysis frameworks to identify discriminative brain connectional features which can be potential quantitative neuroimaging biomarkers for clinical diagnosis.
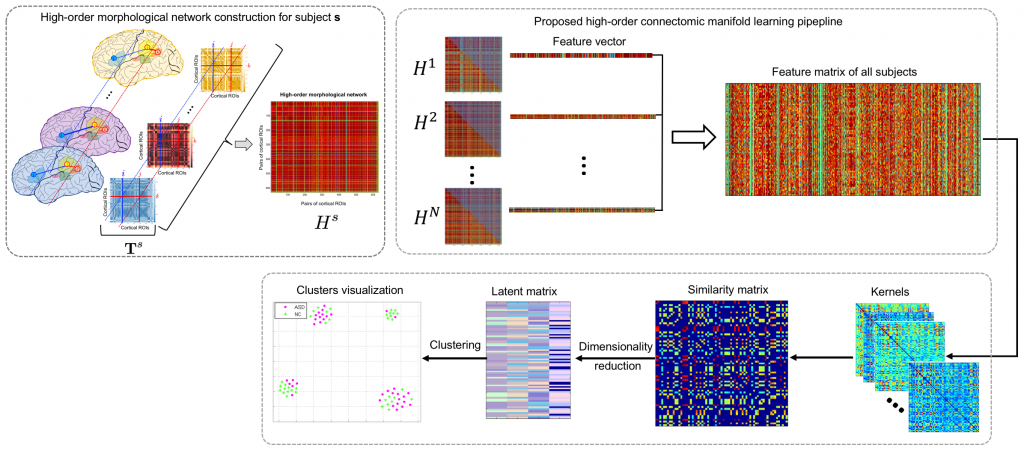